What is DATA SCIENCE? đ
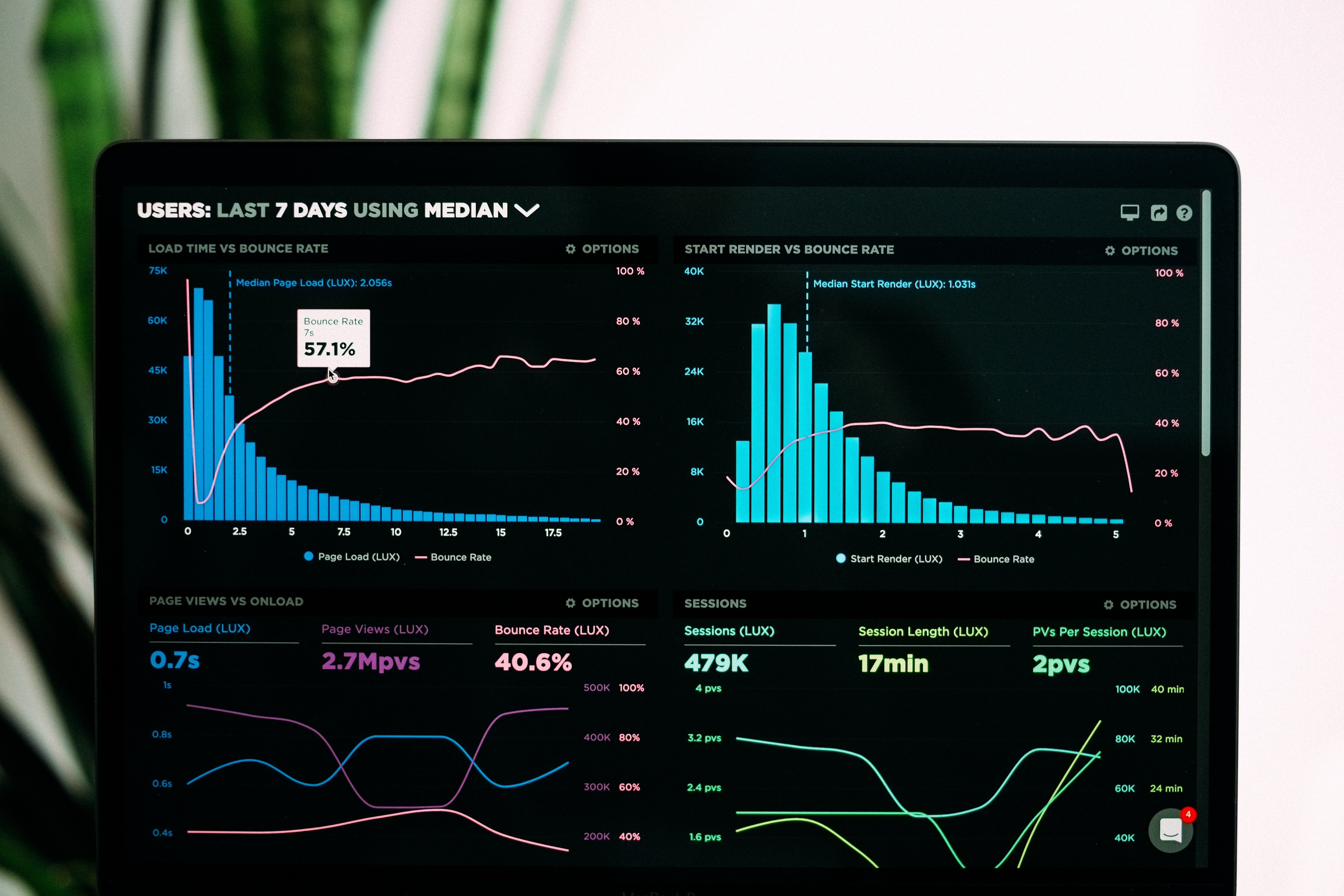
In the era that we are living in, where we are reading posts, sharing interesting moments, and buying cloth on social media and webs, it is well known that the community is feeding a big amount of data into the databases of those companies.
What do I mean by data? When we talk about data, it means facts that are registered and collected for further treatment or analysis. Let's put this in context. Let's suppose that we are looking for some shirts and jeans in Zara. We just choose our favorite clothes for the next season so then, we need to register or log in on the web and then enter our data to continue with the further payment plus delivery. In the end, the companies send us a ticket that has all the information related to our operation. This invoice represents a summary of the data that was collected such as the clothes that were bought, the price, the quantity, the payment method, and so on. This is the real data that the company collects from us to deliver the purchase, and it is ready to be treated and analyzed in the company's systems.
Currently, we are living in a world that is full of data that we leave not only on retail webs or on social media but also in other places like traffic or facility cameras that collect our movements in order to understand and study them. In addition to this, insurance companies could collect telematics data based on devices connected to the car that learn our behavior while we are riding a car to offer personalized products or services. This data is the raw material, the new gold in this age, and is useful to be treated to fully understand customer behavior.
About Data Science
âData Science combines the techniques and methodologies of statistics, mathematics and computer science, with the aim of understanding, interpreting and inferring conclusions from data and making decisions.â
Now that we know what is data and the potential benefits that it has, we will continue to explain the definition of data science. One of the definitions that I learned during my master's degree was that data science deals with the study of data through the scientific method.  Data science combines the techniques and methodologies of statistics, mathematics, and computer science, with the aim of understanding, interpreting, and inferring conclusions from data and making decisions. Â
Based on the definition, we can infer that data science is a multidisciplinary field where domain knowledge, statistics, computer science, and communication skills are used in combination. Furthermore, it is common to listen that data scientists are better statisticians than most programmers and better programmers than most statisticians. Continuing with the definition, we can see that it has different goals like collecting and understanding the data which is called descriptive analytics that answer the question of âWhat happened?â. Another goal is interpreting the data that is part of diagnostic analytics that answer the question of âWhy did this happen?â. In this kind of analytics, we take the analysis a step further, because we are comparing coexisting trends or movements, uncovering correlations between variables, and determining causal relationships where possible. Finally, it talks about inferring conclusions that we can relate with predictive analytics that is used to make predictions about future trends or events and answers the question, âWhat might happen in the future?â
Without data, people make decisions based on biases and beliefs. So, in order to create value and take decisions with data fundamentals, companies use their data, explore it, analyze and predict future behaviors with them. In this scenario, data scientist takes place in order to extract the data, understand it and apply different techniques to solve problems. But, how do they deliver value to the organization? It is by the following goals:
Revenue increase: data science can be applied to find and refine a target customer base to generate more revenue. In sales, specifically, lead management, models can analyze past customers and score leads, resulting in greater sales efficiency. Not only past in-house customer data is used, but also social media interaction for scoring. Companies that applied artificial intelligence lead scoring increased by 50% more appointments and a 60% reduction in call time [2]. Better leads close sales deals faster, which creates more revenue.
Cost reduction: In my own personal experience in financial services, we have applied data science concepts to reduce customer turnover and fraud risk. Using predictive models, we predict when customers are likely to get into trouble and offer assistance to prevent breaches of contract. As for fraud prevention, we analyzed client data to detect anomalies that might be fraudulent, which leads to a potential savings of millions per year.
More efficient processes: for example data science applied with real-time reporting [4], customer interactions are more effective by allowing service representatives to better understand consumers while interacting with them. Think about how many times youâve made a call to a customer service line and waited on hold only to answer a bunch of questions, be put on hold again, and then have to answer all the same questions with everyone else you talk to. With real-time reporting, customer service agents have instant access to the questions callers have already answered, shortening the call and hold times while improving the overall experience.
Knowledge discovery: it is related to the extraction of knowledge of the data collected that was previously unknown, and it has useful information
More efficient decision-making: With the help of data science, decision-makers are now able to make better-informed choices than in the past by shaping and filtering the data their organizations have collected. By using this data, they are able to formulate predictions of the future based on what would happen if they decided to take their organization in a brand-new direction, for example, or how they would rebuild themselves after a financial disaster, among a variety of other things. For example, in Airbnb, the marketing department thought one of their campaigns was off due to bad metrics [3]. A data scientist found the root cause to be a group in Asia using their site to browse neighborhoods. As a result, the marketing department redirected that group to a travel site, which clarified their marketing metrics and saved resources instead of launching an inefficient marketing initiative.
References
[1] Lester Leong, How Data Science makes money for businesses (2019), https://towardsdatascience.com/how-data-science-makes-money-for-businesses-203daba010c1
[2] T. Capone, How Data Science Can Help Your Enterprise Generate More Revenue (2018), https://conceptainc.com/blog/how-data-science-can-help-your-enterprise-generate-more-revenue/
[3] N. Patel, How Airbnb Uses Data Science to Improve Their Product and Marketing (2019), https://neilpatel.com/blog/how-airbnb-uses-data-science/
[4] Landon Starr, How Data Science Can Improve Business Efficiency (2018), https://blogs.oracle.com/ai-and-datascience/post/how-data-science-can-improve-business-efficiency